Machines Learning and Facies Discrimination; Preliminary Results
Machines Learning


DOI:
https://doi.org/10.70447/ktve.2322Keywords:
machine learning, gradient boosting, well logsAbstract
Artificial intelligence and machine learning applications have been used to distinguish the geological sequence from well logs. A tree-based training model was developed using the reinforced derivative algorithm, one of the consultative machine learning method classifiers, to predict facies based on well log data, and improvements were made on the data collection to increase the prediction success rate. As the data collection, well data around Kansas (USA), recommended for machine learning by the Society of exploration geophysics, was used. In the study, well logs were introduced, the reinforced derivative classifier algorithm as a machine learning method and the classification of data were explained, and prediction results were obtained with 57% accuracy on the trial well and 88% accuracy with adjacent facies information
Downloads
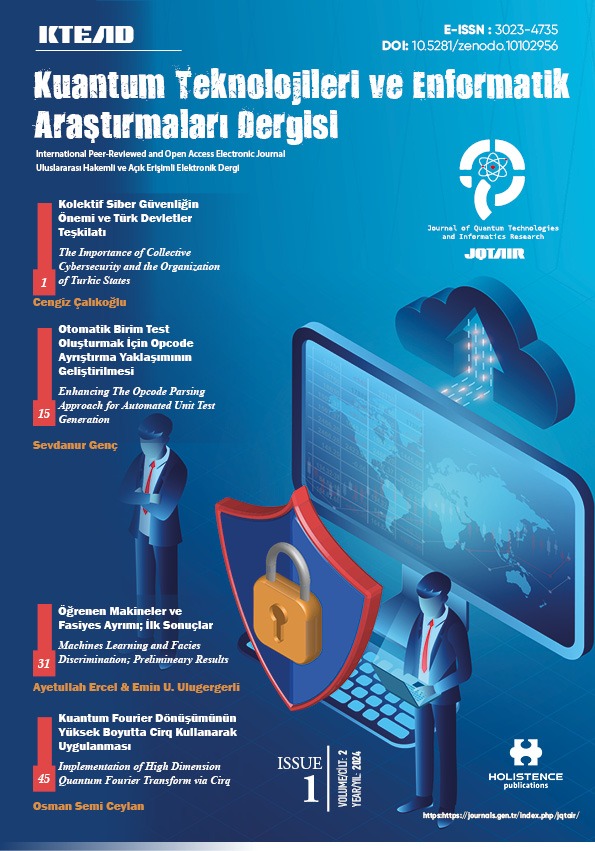
Downloads
Published
How to Cite
Issue
Section
Categories
License
Copyright (c) 2024 Ayetullah- Emin ULUGERGERLİ

This work is licensed under a Creative Commons Attribution 4.0 International License.
Key Points of CC BY 4.0
- Attribution Requirement: Others can share, adapt, and use the work, even commercially, as long as they credit the original author(s) and the journal (JQTAIR) as the source.
- Flexibility in Usage: This license maximizes dissemination, as anyone can use the research in new projects, derivative works, or even commercial applications.
- Global and Broad: The 4.0 version is legally global, compatible with other open-access content, and widely accepted by institutions and funders worldwide.
- Freedom to Remix and Adapt: Researchers, educators, and industry professionals can freely build upon the work, encouraging collaboration and innovative uses in various fields.
Attribution Requirement for Users
To comply with CC BY 4.0, anyone who uses, shares, or builds upon the journal’s work must include:
- The title of the work.
- A link to the full text (ideally hosted on JQTAIR’s site or repository).
- Credit to the original authors and the journal (e.g., “Published by JQTAIR” or “Original work by [Author Name], published in JQTAIR”).